Bridging research and education: Dr. Zhang’s dynamic integration of machine learning
Bridging research and education: Dr. Zhang's dynamic integration of machine learning
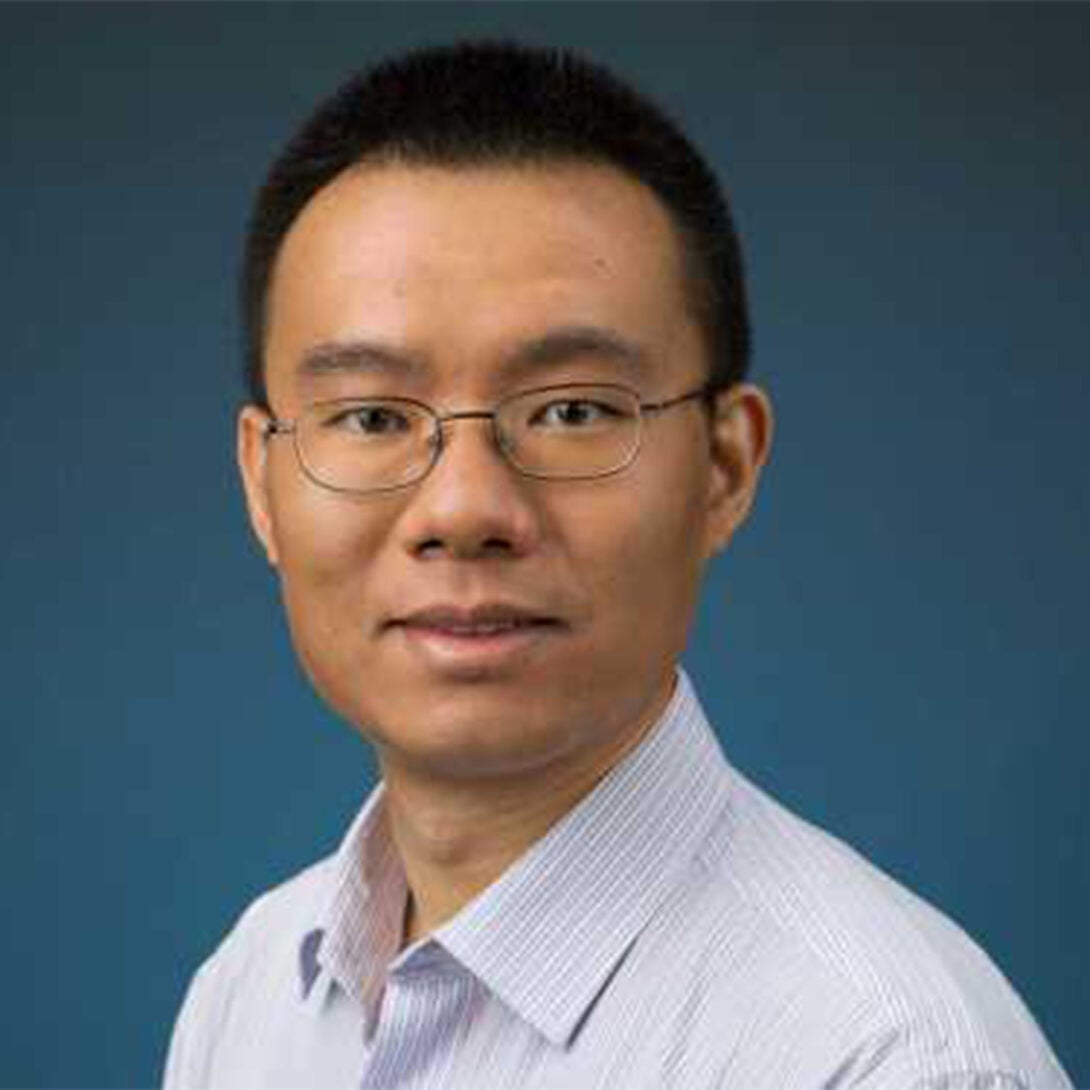
Dr. Xinhua Zhang, an Associate Professor in the College of Engineering at the University of Illinois Chicago (UIC), is a driving force at the intersection of theory and practice. Prior to joining UIC in 2015, Dr. Zhang was a Senior Researcher at the Machine Learning Research Group of National ICT Australia (NICTA, now Data61), as well as a NICTA-endorsed PhD student in the Research School of Computer Science at the Australian National University.
Dr. Zhang teaches UIC’s online Master of Engineering with a focus area in AI and Machine Learning CS 412: Intro to Machine Learning course. He leverages his expertise by guiding students to formulate and solve predictive data analysis problems, selecting appropriate machine learning techniques, and evaluating performance metrics.
Recently, Dr. Zhang led a groundbreaking National Science Foundation (NSF) funded project titled “Learning Generalized Invariant Representations in Banach Space for Transfer Learning” which tackles complex challenges in domains such as computer vision and natural language processing. Through research and collaboration with fellow academics and Ph.D. students, the project yielded significant insights and practical applications.
Learn more about Dr. Zhang’s recent NSF-funded research project and why he encourages his students to get involved in research.
Tell us a little about yourself.
I became interested in computer science because it strikes a good balance between theory, especially mathematics, and practice. It allows nontrivial theoretical concepts and tools to be put into everyday use that directly benefits human life.
Compared with other engineering domains, computer science has a relatively lower entry threshold. When I was a student, one only needed a computer to get started, along with Internet connection. In comparison, there was so much one can do on it, such as games, solving mathematical problems/puzzles, learning foreign languages, etc. At a more fundamental level, the problem-solving techniques and principles of computing are particularly fascinating. How to abstract and formulate a real-world problem in a way that is amenable to a computer to solve (as opposed to a human’s way), and how to verify the correctness of the solution under the restrictions of computing resources. Learning these techniques changed my mind of analyzing and solving real problems in my life.
Can you tell us about the NSF-project you were recently a principal investigator (PI) for? What was your experience like?
I have recently completed an NSF-funded project, titled ‘Learning Generalized Invariant Representations in Banach Space for Transfer Learning.’ It was funded for four years by the Robust Intelligence program within NSF’s Directorate for Computer and Information Science and Engineering (CISE). Humans often reason about their observations abstractly to prevent themselves from drawing incorrect conclusions based on unimportant differences. For example, a person tries to avoid on-coming traffic regardless of the lighting conditions; in this case, illumination is said to be an invariance to the problem of traffic avoidance. The goal of this project is to create methods and algorithms to make decisions by learning representations of data with invariances. Specifically, it applies linear and sublinear regularizations on a reproducing kernel Hilbert space to introduce invariant representations in the resulting Hilbert or Banach spaces. The project discovered that the method could model far more delicate invariances than “rotating an image does not change whether it shows a cat”. In fact, it found new methods to learn more flexible forms of invariances. The result benefits a wide range of social and real-world applications including computer vision, natural language processing, and graph-structured data analysis.
The experience of this NSF-funded research was very challenging but rewarding. I really enjoyed working with the co-PI Professor Brian Ziebart and our Ph.D. students. Through this journey, we were able to resolve technical challenges and identify further impactful directions for research. We also presented the papers at top conferences of machine learning such as the International Conference on Machine Learning (ICML) and Conference on Neural Information Processing Systems (NEUR IPS).
Can you tell us about the process of applying for NSF-funded grants?
NSF releases solicitations for proposals, including both ongoing programs and special interest programs with specific deadlines. The solicitation will specify their area of interest, funding level, scope, and eligibility. The most important component when applying is a proposal. The project description generally spans 15 pages and needs to describe what problem is to be addressed, why it is important, what new technology will be proposed, how to evaluate the success, what alternative solutions are available, why the budget is appropriate, and how the research will be integrated into education and outreaching.
For ML-oriented proposals, one will also need to provide a project summary, data management plan, year-to-year budget, bio-sketches of the senior personnel, conflict of interest, facilities and resources available, and statement of diversity, equity, and inclusion. Many ML proposals involve collaborations of researchers from application and methodology, so it is important to form a team. Once submitted, the proposal will be reviewed by a panel.
Are your students able to get involved in your research projects? Do you use your projects as examples in your online MEng courses?
I have used another NSF-funded project as an example in my online MEng course. I am a co-PI of the NSF grant “Addressing the 3D Challenges for Data-Driven Fairness: Deficiency, Dynamics, and Disagreement”. In this project, I develop fair machine learning approaches based on robust estimation to address the deficiency of information. As an essential part of machine learning, fairness has also been seamlessly integrated in our MEng courses. In my MEng 412 course, students have an opportunity to develop new risk functions that promote fairness.
My Ph.D. students have also been heavily involved in research projects and have been making significant contributions in formulating new ideas, developing them into concrete and efficient algorithms, and conducting experiments to demonstrate their empirical effectiveness.
Why would you recommend a MEng student get involved in research? Why is it a worthy career path?
Getting involved in research can significantly deepen one’s understanding of cutting-edge machine learning techniques, from both a theoretical and an applied perspective. It also trains students in identifying potentially impactful and promising research directions, a skill that is useful even if one does not pursue a lifelong career in research. ML is now rapidly expanding its frontier, presenting many fruitful research opportunities that will make the world a better place.